Prescriptive analytics is the use of the descriptive, predictive, and human elements of analytics to inform business decisions.Prescriptive analytics can cut through the clutter of immediate uncertainty and changing conditions. It can help prevent fraud, limit risk, increase efficiency, meet business goals, and create more loyal customers.
Is prescriptive analytics difficult : Because prescriptive analytics is the most complex type of data analytics to get right, it's also the most error-prone. It requires a complex combination of technical, communication, and business skills, explaining why there is currently a surge in demand for data analysts with this kind of expertise.
What are the pros and cons of prescriptive analytics
Pros and Cons of Prescriptive Analytics
With prescriptive analytics, you can make fast, data-based decisions that are designed to reduce your risk. In turn, this can make your operation more efficient as a lot of the leg work is done for you. The downside, of course, is that the data is only as good as what it's fed.
What are the merits and limitations of prescriptive analytics : Disadvantages of Prescriptive Analytics
It requires a large amount of data.
The required computing power is high.
It can take a long time to implement prescriptive analytics.
Not always accurate.
Challenges and Limitations of Prescriptive Analytics
Data Quality and Availability: Prescriptive analytics heavily relies on high-quality data. Inaccurate, incomplete, or outdated data can lead to unreliable recommendations. Ensuring data accuracy and availability is a persistent challenge. Predictive models can perpetuate existing biases and discrimination if they are trained on biased data. Additionally, predictive analytics can raise privacy concerns if personal data is used without consent or is shared with third parties.
What is the impact of prescriptive analytics
Prescriptive analytics plays a pivotal role in enhancing business decision-making by providing valuable insights and actionable recommendations. One key contribution lies in its ability to optimize choices.One of the most significant limitations of predictive analytics is data quality. Predictive models rely on large, accurate, and relevant datasets to produce accurate predictions. If the data used to train the model is incomplete, inaccurate, or biased, the model's predictions will also be flawed.This analytics can help businesses make better decisions based on the data they have. We can use prescriptive analytics for many things, including predicting customer behavior, optimizing marketing campaigns, and improving supply chains. However, predictive analytics also comes with some risks, such as data privacy, bias, overfitting, and false positives. How can you avoid these risks and make the most of predictive analytics for software quality
What are two limitations of predictive analytics : Predictive Analytics is Limited in Value Due to the Way Decision Makers Respond
Hypothesis-driven responses that rely heavily on best practices, gut feel, and spreadsheets.
Data-driven responses that rely heavily on optimization models.
What are the limitations of data in predictive analytics : Incompleteness. The accuracy of predictive analytics models is limited by the completeness and accuracy of the data being used. Because the analytical algorithms attempt to build models based on the available data, deficiencies in the data may lead to deficiencies in the model.
What is the limitation of a predictive study
Predictive models optimize product design but have limitations. They rely on historical data, risking inaccuracy with changing conditions and biases. Overfitting and underfitting impact performance, and understanding causation is challenging. Ethical concerns arise from biased data.
Antwort What are the limitations of prescriptive analytics? Weitere Antworten – What are the disadvantages of prescriptive analytics
Disadvantages:
Prescriptive analytics is the use of the descriptive, predictive, and human elements of analytics to inform business decisions.Prescriptive analytics can cut through the clutter of immediate uncertainty and changing conditions. It can help prevent fraud, limit risk, increase efficiency, meet business goals, and create more loyal customers.
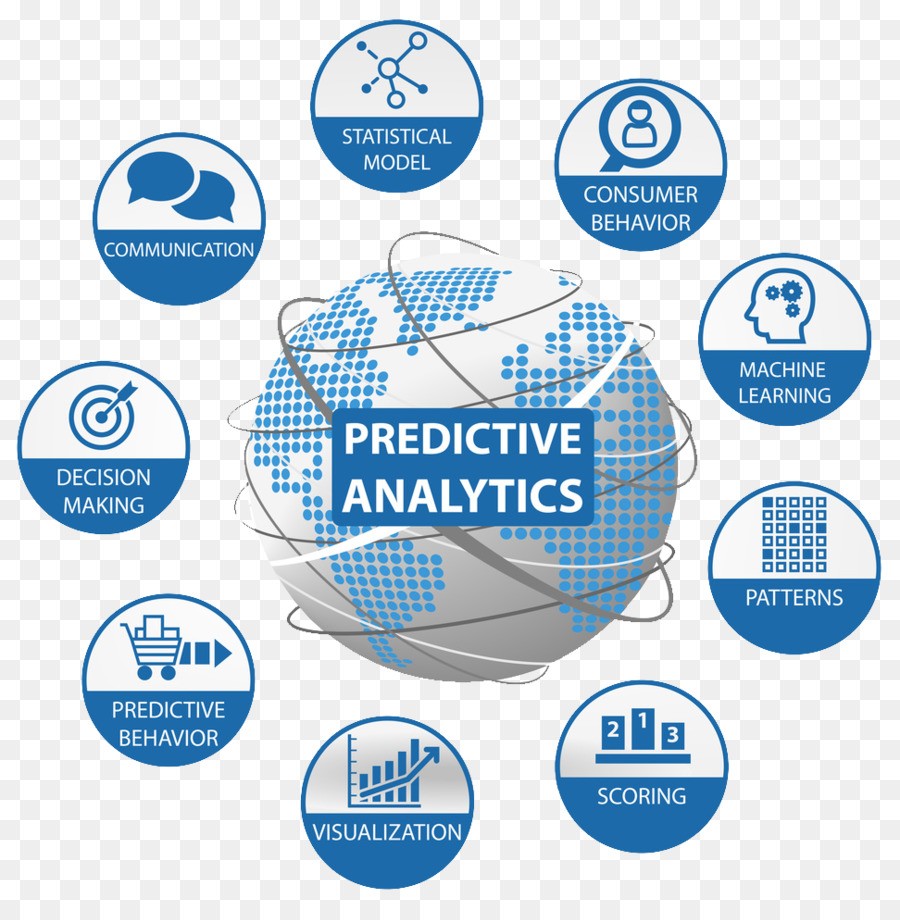
Is prescriptive analytics difficult : Because prescriptive analytics is the most complex type of data analytics to get right, it's also the most error-prone. It requires a complex combination of technical, communication, and business skills, explaining why there is currently a surge in demand for data analysts with this kind of expertise.
What are the pros and cons of prescriptive analytics
Pros and Cons of Prescriptive Analytics
With prescriptive analytics, you can make fast, data-based decisions that are designed to reduce your risk. In turn, this can make your operation more efficient as a lot of the leg work is done for you. The downside, of course, is that the data is only as good as what it's fed.
What are the merits and limitations of prescriptive analytics : Disadvantages of Prescriptive Analytics
Challenges and Limitations of Prescriptive Analytics
Data Quality and Availability: Prescriptive analytics heavily relies on high-quality data. Inaccurate, incomplete, or outdated data can lead to unreliable recommendations. Ensuring data accuracy and availability is a persistent challenge.
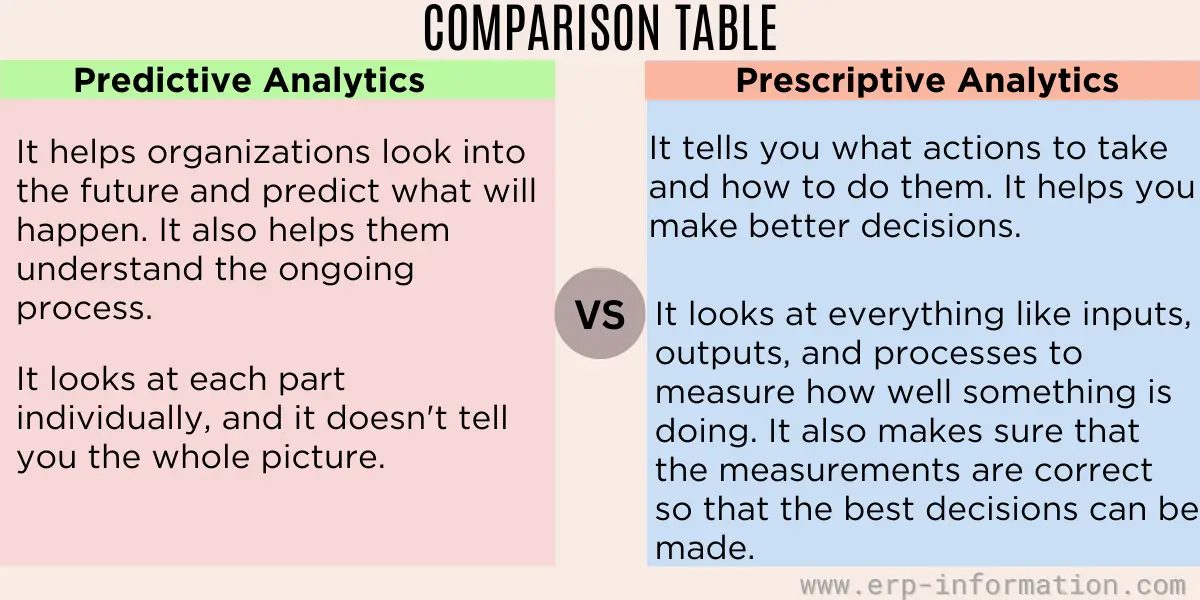
Predictive models can perpetuate existing biases and discrimination if they are trained on biased data. Additionally, predictive analytics can raise privacy concerns if personal data is used without consent or is shared with third parties.
What is the impact of prescriptive analytics
Prescriptive analytics plays a pivotal role in enhancing business decision-making by providing valuable insights and actionable recommendations. One key contribution lies in its ability to optimize choices.One of the most significant limitations of predictive analytics is data quality. Predictive models rely on large, accurate, and relevant datasets to produce accurate predictions. If the data used to train the model is incomplete, inaccurate, or biased, the model's predictions will also be flawed.This analytics can help businesses make better decisions based on the data they have. We can use prescriptive analytics for many things, including predicting customer behavior, optimizing marketing campaigns, and improving supply chains.

However, predictive analytics also comes with some risks, such as data privacy, bias, overfitting, and false positives. How can you avoid these risks and make the most of predictive analytics for software quality
What are two limitations of predictive analytics : Predictive Analytics is Limited in Value Due to the Way Decision Makers Respond
What are the limitations of data in predictive analytics : Incompleteness. The accuracy of predictive analytics models is limited by the completeness and accuracy of the data being used. Because the analytical algorithms attempt to build models based on the available data, deficiencies in the data may lead to deficiencies in the model.
What is the limitation of a predictive study
Predictive models optimize product design but have limitations. They rely on historical data, risking inaccuracy with changing conditions and biases. Overfitting and underfitting impact performance, and understanding causation is challenging. Ethical concerns arise from biased data.